The Simplified Guide: Creating Your AI Model
Welcome to the fascinating world of AI! Today, we're breaking down the seemingly complex process of building an AI model into five easy-to-understand steps. Whether you're a budding enthusiast or just curious about how AI magic happens, you're in the right place.
Step 1: Collecting Data
Imagine teaching a child to recognize animals. You'd probably start with a bunch of pictures, right? That's exactly what we do in AI. The first step is gathering a lot of data related to what we want our AI to learn. For example, if we're creating an AI to identify dogs, we'd start with lots of dog pictures.
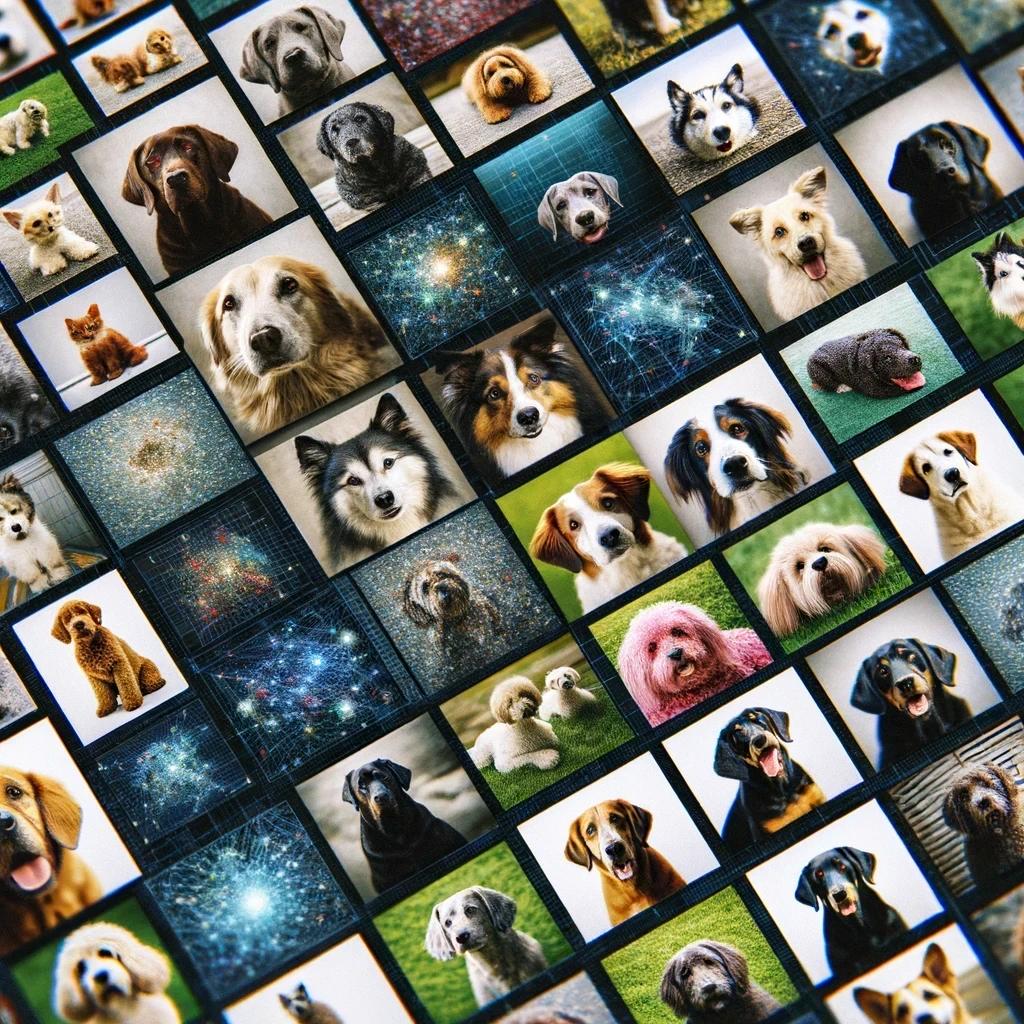
Step 2: Labeling Data
Now, it's not enough to just have the pictures. We need to tell our AI what it's looking at in each one. This is called labeling. If we show it a picture of a Labrador, we label it "Labrador". This helps the AI understand and remember what each thing is.
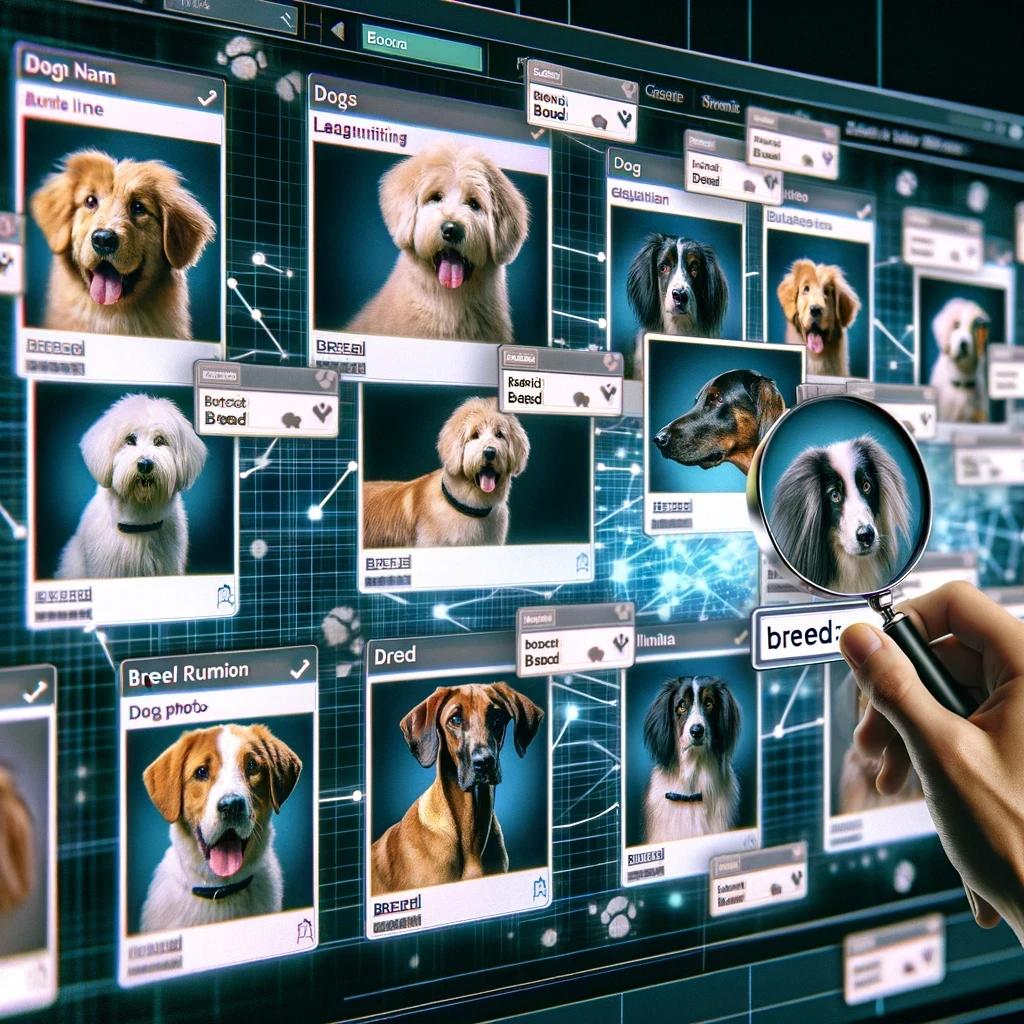
Step 3: Training the Model
Once we have our labeled data, it's time for the actual "learning" part. We use this data to train our AI model. This is like the study phase, where the AI goes through all the data we've given it, trying to understand patterns and differences.
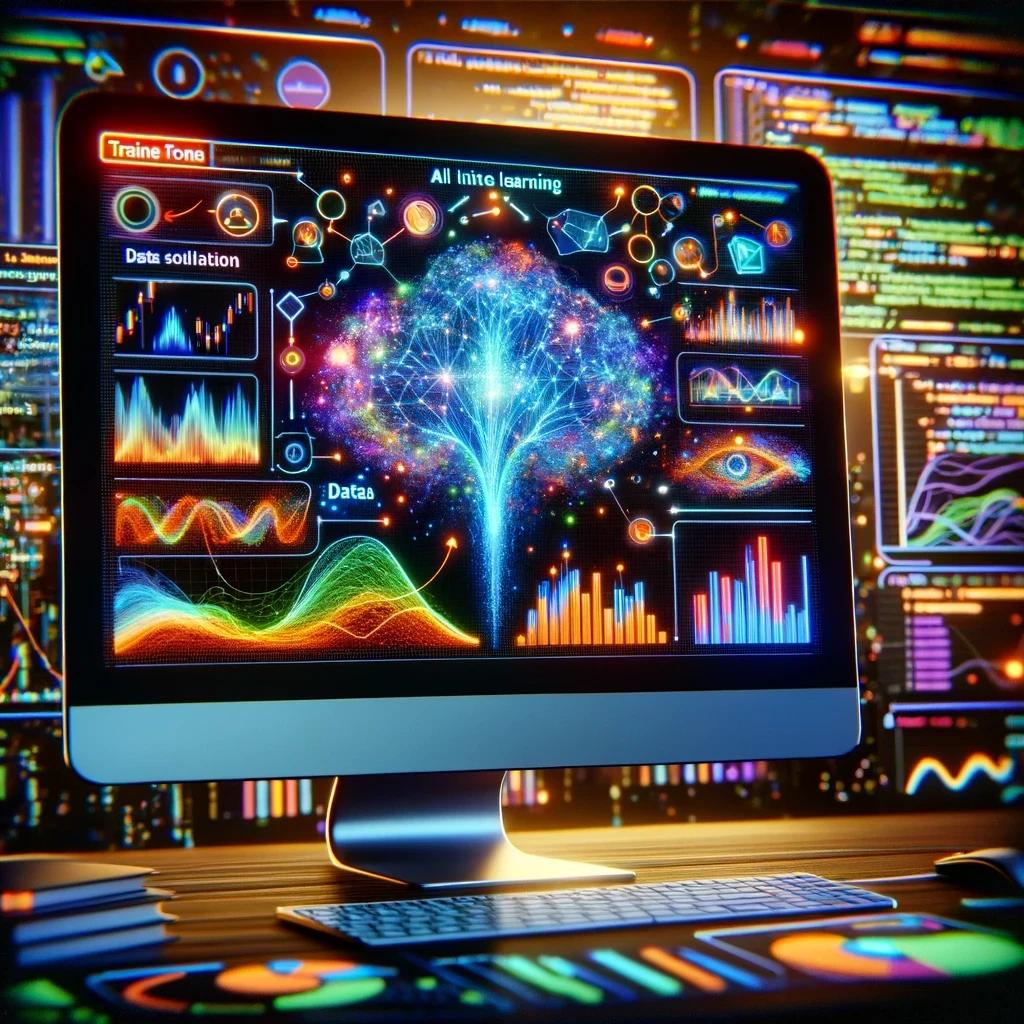
Step 4: Testing and Tweaking
After training, we need to see how well our AI has learned. We test it with new data it hasn't seen before. If it mistakes a cat for a dog, we know we need to adjust things. This step might involve going back to training with more data or tweaking how the AI learns.
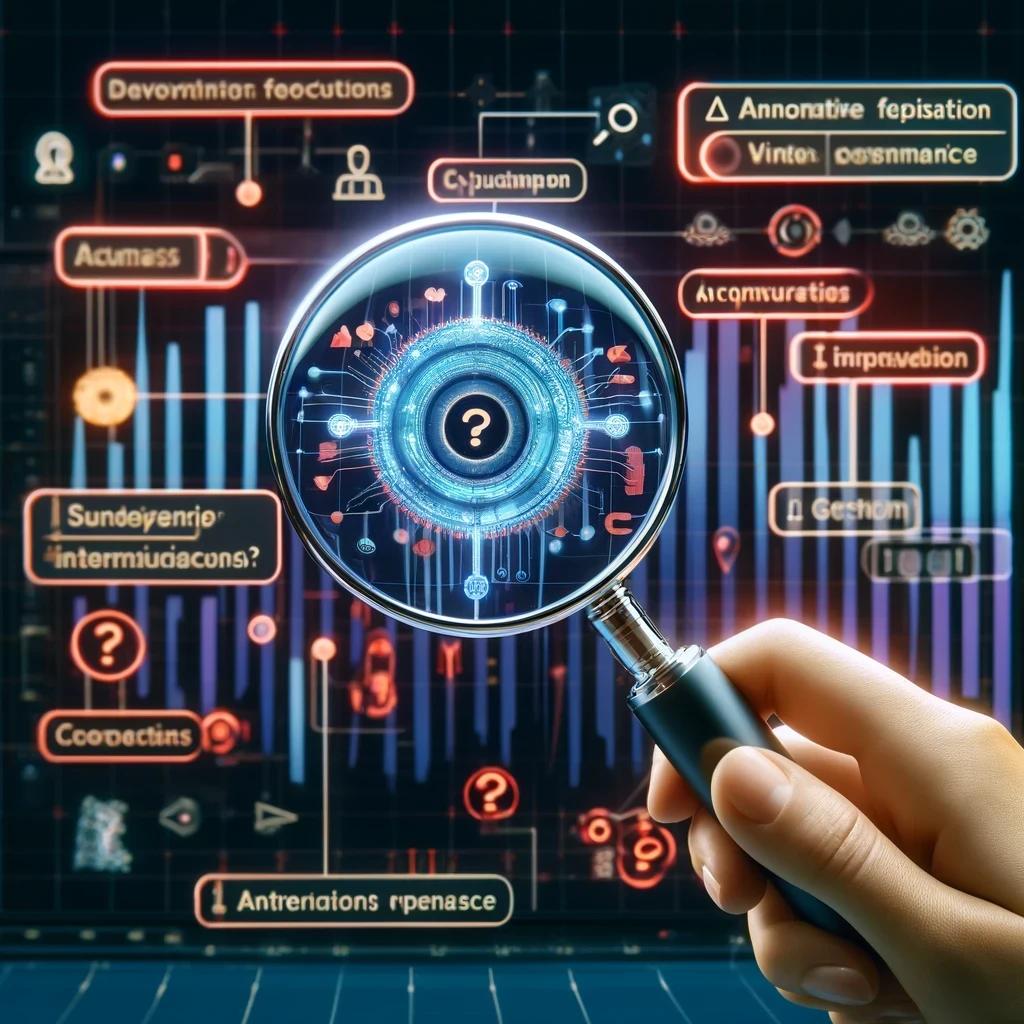
Step 5: Deployment
Once our AI model is smart enough and makes few mis+takes, it's ready to graduate. We deploy it into the real world, where it can start doing its job, like helping answer customer service questions or spotting potential fraud in banking transactions.
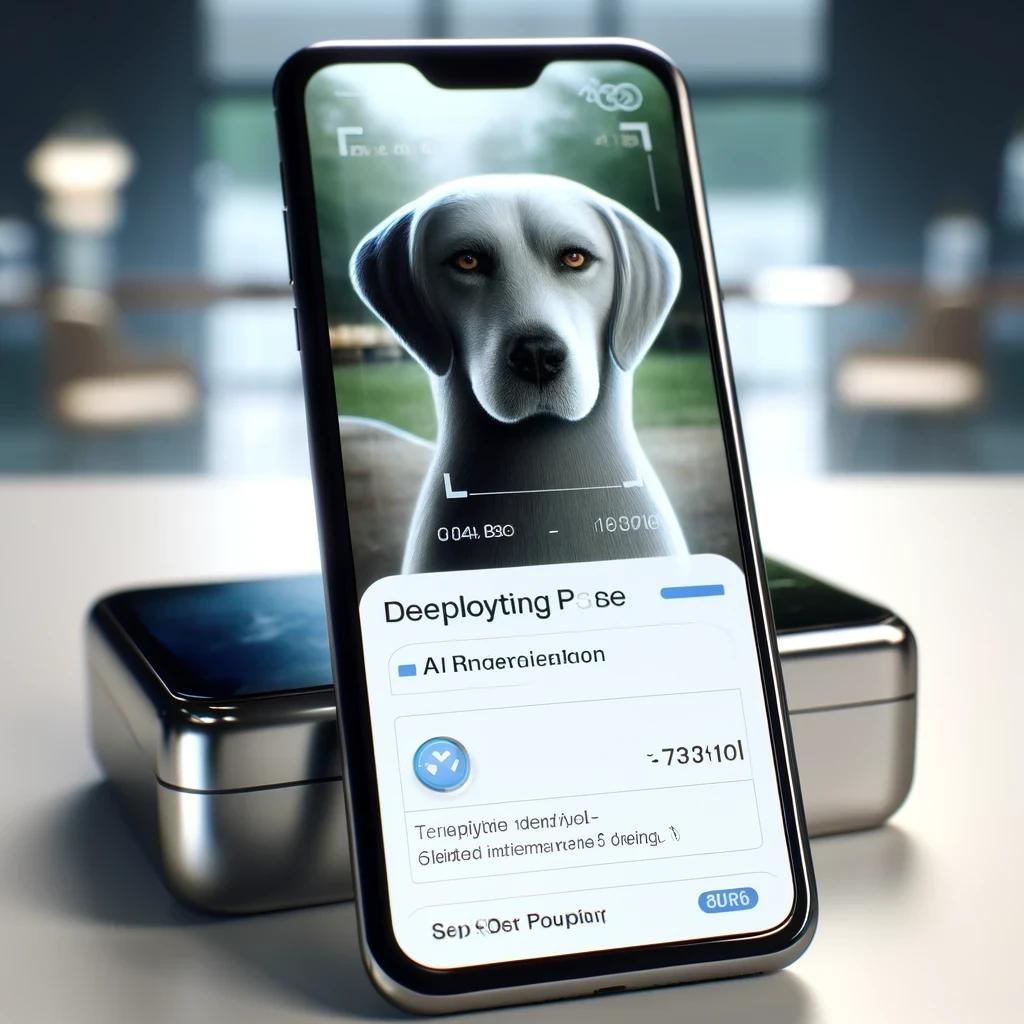
Wrapping Up
Creating an AI model is a bit like raising a smart pet. It needs lots of attention in the form of data, guidance on what's right and wrong, time to learn, checks to ensure it's learning well, and finally, a place to show off its skills. And voilร , that's how you create an AI model, simplified!
Remember, this is a simplified overview. Each step can be a world of its own, full of fascinating challenges and innovative solutions. But don't let that daunt you. Every AI journey starts with a single step, and now you know the first five.