๐ฏ What You'll Learn Today
LangGraph Tutorial: Creating Dynamic Conversation Flows - Unit 1.1 Exercise 4
This tutorial is also available in Google Colab here or for download here
Joint Initiative: This tutorial is part of a collaboration between AI Product Engineer and the Nebius Academy.
This tutorial demonstrates how to build an advanced conversational agent using LangGraph's state management and conditional routing capabilities. We'll create a chatbot that can maintain context, respond appropriately, and gracefully terminate conversations.
Key Concepts Covered
- Advanced State Management with Annotations
- Dynamic Conversation Control
- Conditional Edge Routing
- Message Flow and Termination Logic
Let's break it down step by step:
from typing import Annotated, TypedDict
#!pip langchain-core
from langchain_core.messages import AIMessage, BaseMessage, HumanMessage
#!pip langgraph
from langgraph.graph import END, START, StateGraph
from langgraph.graph.message import add_messages
Step 1: Enhanced State Definition
We define an advanced state structure that combines message handling with conversation control through annotations and flags.
class State(TypedDict):
"""Enhanced state container with message handling and flow control.
This state implementation introduces two key advanced concepts:
1. Annotated message lists for proper LangGraph handling
2. Control flags for conversation flow management
Attributes:
messages: List of conversation messages with LangGraph's add_messages
annotation for proper message management
should_end: Boolean flag to control conversation termination
Note:
The add_messages annotation is crucial for proper message handling
in LangGraph, ensuring correct message propagation through the graph.
"""
messages: Annotated[list[BaseMessage], add_messages]
should_end: bool
Step 2: Conversation Logic Implementation
The conversation node implements our core chat logic with proper state management and flow control.
def conversation_node(state: State) -> dict[str, list[BaseMessage] | bool]:
"""Manages a stateful conversation with controlled flow and termination.
This node demonstrates several advanced LangGraph concepts:
1. State-based decision making
2. Dynamic response generation
3. Conversation flow control
4. Termination management
The conversation follows this flow:
1. Initial greeting
2. Response to greeting
3. Graceful termination
Args:
state: Current conversation state with messages and control flags
Returns:
dict: Updated state containing new messages and termination status
"""
# Initialize conversation if needed
if not state["messages"]:
return {"messages": [HumanMessage(content="Hello!")], "should_end": False}
# Process last message for context
last_message = state["messages"][-1]
# Implement conversation flow logic
if last_message.content == "Hello!":
return {"messages": [AIMessage(content="How are you?")], "should_end": False}
elif last_message.content == "How are you?":
return {
"messages": [HumanMessage(content="Goodbye!")],
"should_end": True,
}
# Handle other cases
return {"messages": [HumanMessage(content="Goodbye!")], "should_end": True}
Step 3: Flow Control Logic
Implement the routing logic that determines conversation continuation.
def should_continue(state: State) -> bool:
"""Controls conversation flow based on state.
This function demonstrates LangGraph's conditional routing capabilities
by examining state to determine next steps.
Args:
state: Current conversation state
Returns:
bool: True to continue conversation, False to terminate
"""
return not state["should_end"]
Step 4: Graph Construction and Configuration
Build and configure our conversational graph with proper routing and conditional edges.
Initialize graph with our enhanced state
graph = StateGraph(State)
Add conversation processing node
graph.add_node("chat", conversation_node)
Configure entry point
graph.add_edge(START, "chat")
Set up conditional routing
graph.add_conditional_edges(
"chat",
should_continue,
{
True: "chat", # Continue conversation
False: END, # Terminate conversation
},
)
Compile for execution
chain = graph.compile()
Step 5: Execution and Testing
Initialize and run our conversational agent.
Set up initial state
initial_state = {"messages": [], "should_end": False}
Execute conversation
final_state = chain.invoke(initial_state)
Display conversation flow
print("Conversation flow:")
for msg in final_state["messages"]:
print(f"Message: {msg.content}")
Key Takeaways
- Advanced State: Combining annotations with control flags enables sophisticated flow
- Dynamic Routing: Conditional edges create flexible conversation paths
- Flow Control: Proper termination handling prevents infinite loops
- Message Management: Annotated messages ensure correct state updates
Common Pitfalls to Avoid
- Forgetting the add_messages annotation
- Not handling all possible message states
- Improper termination condition setup
- Missing edge cases in conversation flow
Next Steps
- Add error handling and recovery
- Implement more sophisticated conversation patterns
- Add conversation history management
- Integrate with external systems
Expected Output
Message: Hello!
Message: How are you?
Message: Goodbye!
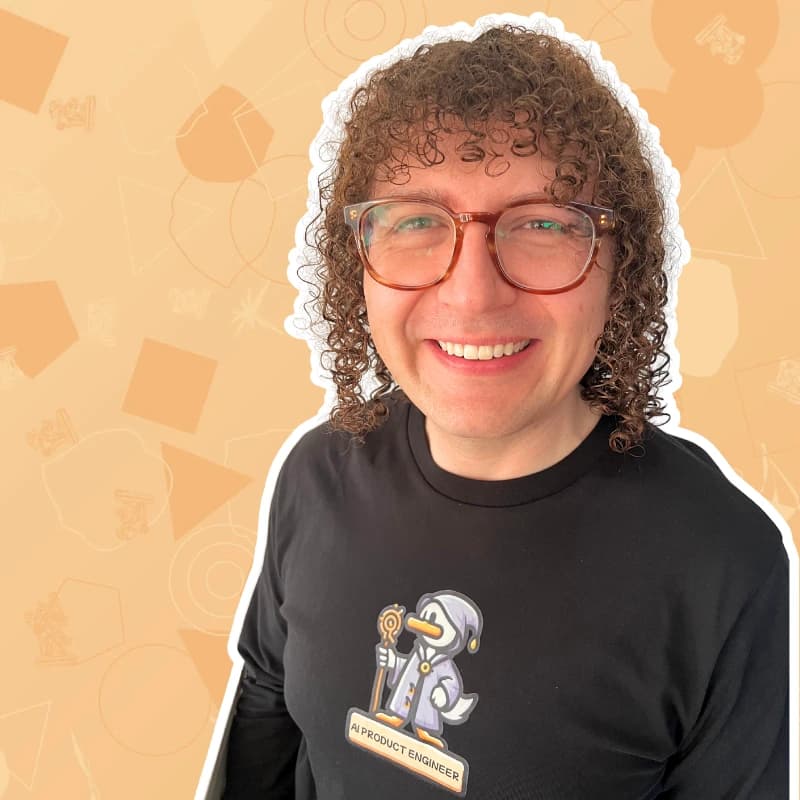
๐ฌ๐ง Chapter