Discussing Langfuse with Max Tee
In this episode, Rod and Max discuss the importance of observability for LLM (Large Language Model) applications and explore the case of Langfuse, an observability platform for LLMs. They examine the market size and investment considerations for Langfuse, highlighting the team's expertise and the potential for growth in the LLM space. The conversation also touches on the benefits of open source software and the challenges of onboarding and pricing in the observability market. They discuss strategies for existing ML observability players to stay relevant in the LLM era and offer advice for building a successful LLM observability company.
Takeaways
- Observability is crucial for LLM applications, and companies like Langfuse provide specific tools to address the unique needs of generative AI.
- Open source software can simplify the onboarding process and attract independent developers and smaller organizations.
- Usage-based pricing offers flexibility and scalability, but companies should also consider the need for predictability and budget planning.
- Existing ML observability players can collaborate with LLM observability companies to expand their offerings and increase their contract value.
- Improving onboarding and providing education are key strategies for LLM observability companies to attract and retain users.
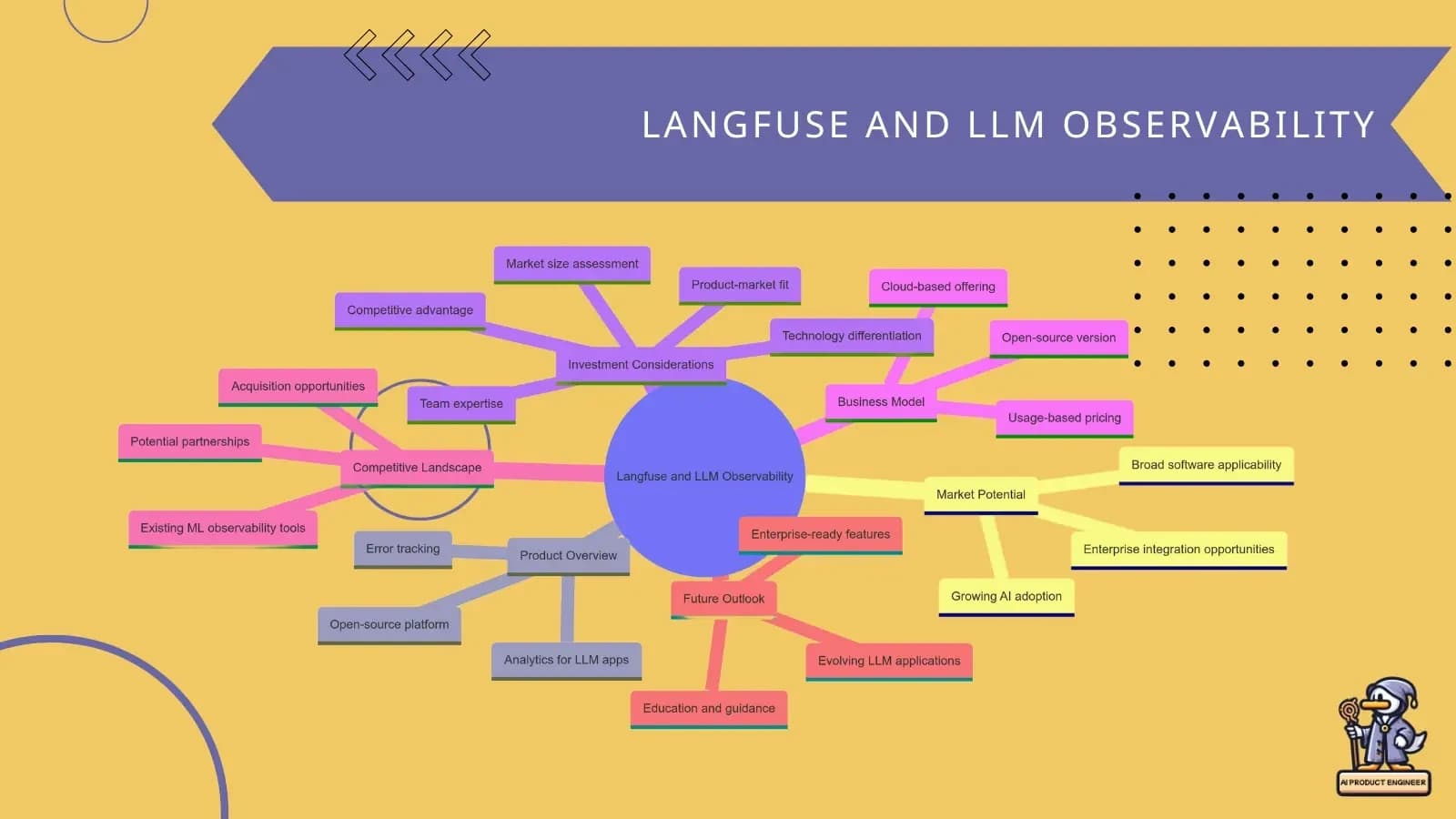
Episode Transcript
Langfuse and LLM Observability
Rod Rivera: Welcome to our first episode of the AI Products podcast. I'm here with Max, a seasoned observer in the VC industry and corporate innovation space. Max, how are you doing?
Maxson Tee: I'm doing well, Rod. Thanks for the introduction, though I'm not sure about the "veteran" part - it makes me sound old! But I'm excited to be here and learn more about AI with you.
Rod Rivera: Absolutely! Our goal with this series is to explore how AI products are built, not just from a technical standpoint, but also as businesses. We want to understand what it takes to build an AI application or product.
Maxson Tee: That's exactly what I'm keen to learn about too. Coming from a non-technical background, I'm eager to understand what's happening under the hood. We can break it down and try to understand what sets some of the top AI companies apart. We've all heard the buzzwords about AI taking over the world, but the real question is: how?
Introducing Langfuse
Rod Rivera: Indeed. This week, we're diving deep into Langfuse, an LLM observability platform. For those who aren't familiar, Langfuse offers an open-source product suite that allows anyone building LLM applications to perform analytics and keep track of errors and other issues with their LLM apps. Max, what are your thoughts on Langfuse?
Maxson Tee: Langfuse is fascinating from my perspective. Since the ChatGPT craze, virtually every large corporation has been looking into Generative AI, and suddenly every startup became an LLM user. The ability to observe different large language models and how they're performing, similar to how you'd normally observe your application, is incredibly interesting. The market potential is huge - if AI can be applied to any software out there, your market is essentially as big as the software industry itself, and possibly even beyond that.
The Need for LLM Observability
Rod Rivera: What I find intriguing is that observability for machine learning isn't new. There have been successful products for classic observability across all types of applications, and specifically for machine learning models in the open-source space, like Evidently AI. Now we're seeing a new wave of entrants like Langfuse, who argue that for LLMs and generative AI, we need a different set of tools. They're saying this is adjacent to, but separate from, the existing market for classic machine learning observability.
Maxson Tee: You're right that it's not entirely new, which is important. The need to observe what's running, how it's running, and whether it's performing well applies to applications, machine learning models, and now LLMs. With the advent of LLMs, many of the tools you'd normally deploy on an application and machine learning would also apply to LLMs. So the same problem sets still exist, even though the underlying models are different. There's a fundamental need here that I think is important - no matter where you go, you always need to observe what's going on. It's a bit like watching the weather, in a way.
Investment Considerations
Rod Rivera: If someone wants to build a company in this space, what would you look for as an investor? What would be the main questions you'd ask to make an investment decision for a company like Langfuse?
Maxson Tee: That's a great question. Given that the LLM space is exploding at the moment, there are several considerations:
- The team: Based on what I've seen, Langfuse's team is impressive - young and trying to do something different.
- Market size: As we discussed earlier, I believe the market could be quite substantial.
- Technology differentiation: Understanding how unique their technology is.
- Market readiness: Are people actually buying the solution? Is there a real need, and if so, how soon will companies be ready to adopt it?
- Competitive advantage: What's their moat? How would Langfuse stand out if new entrants or alternative players come in?
These would be my top five categories to consider.
Team and Product
Rod Rivera: Let's dive deeper into each of those. You mentioned liking the team, and we know they got into Y Combinator and have big-name investors backing them. Why do you think these prominent investors are betting on Langfuse?
Maxson Tee: Based on their Product Hunt launches and other information, it seems Langfuse is solving a real need. There's definitely a ride-the-wave aspect with LLMs, as everyone from corporations to startups is exploring Gen.AI use cases.
What I find interesting about the team is their ability to pivot. You mentioned that when they entered YC, they came with a different idea but quickly realized there was an opportunity to do something else. That shows me they're a team that knows when to change direction and has the courage and ability to do so. It suggests they're doers and potentially quick leaders in this space.
Rod Rivera: I've actually used Langfuse in the past, and while it's a new product with some rough edges, what impressed me was how quickly the team reacted. They answered all my questions and fixed issues promptly. Their responsiveness and flexibility were remarkable.
Product Advantages
Maxson Tee: That's great to hear. What made you choose Langfuse over other solutions out there?
Rod Rivera: What I like is that it's open source, which makes it simple to test without needing to talk to a sales representative. You can just download and install it. I also appreciate their documentation, which is often lacking in open-source products. Their GitHub repository shows active development and community interest, with around 1,800 stars. This demonstrates that if I invest time in this product, I'll get something out of it.
Maxson Tee: That's interesting. The open-source model gives you visibility into their development activity, which you wouldn't have with a closed-source solution. It's a great way to build trust and show potential users that the product is actively maintained and improved.
Open Source vs. Closed Source
Rod Rivera: Exactly. But it's worth noting that some successful products in this space, like DataDog, are not open source yet still make it easy for developers to get started. The key is how easy it is to put the product in the hands of the user. Open source can be great, but only if it's well-documented and easy to set up. Otherwise, companies might prefer a paid solution that offers a smoother onboarding experience.
Maxson Tee: That's a crucial point. It reminds me of decisions we had to make in enterprise blockchain back in 2017. Sometimes, having professional support and ease of deployment can outweigh the benefits of open source, especially for large corporations.
Business Model and Pricing
Rod Rivera: Let's talk about Langfuse's business model. They offer both an open-source version and a cloud version. How do you see this dual approach?
Maxson Tee: It's an interesting strategy. They're targeting different markets with their deployment models. The open-source version allows people to try it out freely, while the cloud version offers a scalable, managed solution with a freemium model. It's almost like they're following the Slack model - let people use it for free, then charge per seat as usage grows.
Rod Rivera: Yes, they can leverage the trend of usage-based pricing, which is attractive because it's pay-as-you-go. However, my experience with enterprise customers is that they often prefer predictable budgeting. A mixed model could work well here - a fixed component for the web interface, perhaps charged per seat, plus a variable component based on usage.
Market Size and Potential
Rod Rivera: Let's discuss potential market size. One way to look at it might be to consider DataDog, which is valued at around 40 billion market. Does that seem reasonable?
Maxson Tee: It's hard to say precisely, but I think it will be at least a billion-dollar market. Every step in software development - from basic automation to machine learning to LLMs - requires some form of observability. The current speculation is that everything can be LLM-fied. The question is how much of that will actually happen.
To get a more accurate estimate, we'd need to dive into how many LLM applications are being deployed, which types of applications would use this, and how much companies are willing to pay for observability. We'd also need to look at enterprise software budgets and estimate what percentage might be allocated to LLM-related tools.
Advice for Langfuse and Competitors
Rod Rivera: What advice would you give to anyone wanting to build a Langfuse competitor, or to Langfuse themselves?
Maxson Tee: While I'm not sure I'm qualified to give advice, I think Langfuse is doing well with their open-source approach. For selling to large enterprises, they'll need to ensure they're enterprise-ready - things like easy installation, SSO capabilities, and other features that big companies expect.
Rod Rivera: From a product perspective, I think there's always room to improve onboarding and make adoption easier. Also, given how early we are in the generative AI journey, providing education and guidance to users could help Langfuse or other players in this space strengthen their market position.
Maxson Tee: Absolutely. As LLM applications become more prevalent, tools like Langfuse will become increasingly important. As one of the Kosla Ventures partners said, "Chase value, not valuation." That's a good principle to keep in mind in this rapidly evolving space.
Conclusion
Rod Rivera: Well, Max, this has been a fantastic discussion. Let's continue next week with our next company to assess, analyze, and explore.
Maxson Tee: Perfect. We're here to learn, and for all the listeners out there, if there's anything you want us to talk more about, give us a shout.
Rod Rivera: Exactly, Max. Until next week!